The data we need to end poverty by 2030
We need quality gender data if we are serious about our commitment to ‘leave no one behind’.
For the first time in decades, poverty has increased globally.
The World Bank estimated that COVID-19 would push 150 million people into extreme poverty by the end of 2021. For the individuals who were already experiencing poverty and inequality, COVID-19 has deepened it.
Women have been unevenly and unfairly impacted by the pandemic and its effects, with UN Women estimating that an additional 47 million women and girls will fall into extreme poverty this year alone. Meanwhile, no country is on track to achieve gender equality anytime soon. At the current rate of progress it will take 135 years to close the gender gap (that’s another two generations away)! Progress is not just stalling, we are actually going backwards. Gains that have been made over recent years are being eroded and undone.
This the backdrop against which the global community is working to achieve the 2030 Agenda for Sustainable Development – the shared blueprint for peace and prosperity for people and the planet. The 17 Sustainable Development Goals (SDGs) are at the heart of this agenda, but (spoiler alter) we’re behind on achieving them and time is running out. We now have less than nine years to end poverty in all its forms, achieve gender equality and empower all women and girls, and reduce inequalities within and among countries (just 3 of the 17 goals).
This week, the High Level Political Forum (HLPF) is taking place. As the central platform to follow up and track progress towards the SDGs, HLPF is an important part of the 2030 Agenda and its promise to ‘Leave No One Behind’. It is also an opportunity to identify the areas where we need to roll up our sleeves, and take action so that we can speed up progress. Every year, a handful of goals are selected for review and Goals 1 – No Poverty and Goal 10 – Reduce Inequalities are two of the goals under review this year.
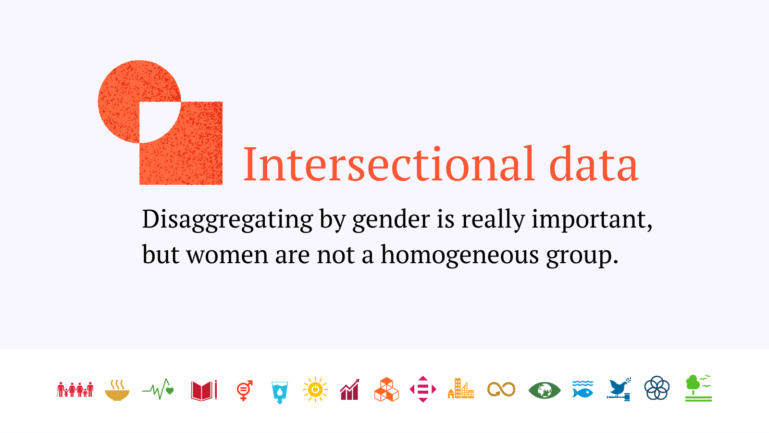
The problem with current poverty and inequality measurements
Even before COVID-19, the world was off track to end poverty by 2030. So what can we do to get back on track? As a starting point, we need to collect more and better quality data.
The way we are measuring poverty and inequality is problematic. Right now, policies and decisions to take action on the SDGs are being made using poverty data that hides the experience of half the population (women). To design gender-responsive, effective and efficient policies and programs that reduce poverty and inequality, policy-makers need to have data about different areas of people’s lives so that they can have the greatest impact. But the data we need to make policies, programs and services that address the root causes of poverty are inadequate at best, and non-existent at worse.
Global poverty data should be able to tell us basic information about the lives and experiences of individuals who experience poverty and inequality – things like how does gender, age, ethnicity, ability, sexuality, religion, geography or the intersection of these factors create, shape and compound experiences of poverty and inequality. But actually, it can’t. Global poverty data can’t show us if people who experience poverty more likely to be men, women, older, or younger.
The reason for this is that in the majority of cases, poverty continues to assess the circumstances of households, not individuals. Changing this was identified as a priority in 1995 at the Fourth World Conference on Women in Beijing, which emphasised the importance of investing in and collecting ‘gender and age-disaggregated data on poverty’. More recently, it was reiterated as a gap in the 2017 report of the World Bank’s Commission on Global Poverty.
A generation later, the implications of this mean poverty data still cannot be accurately disaggregated to show how experiences of poverty are influenced by gender, age, ability, or intersections of these despite evidence that these factors create, shape or deepen experiences of poverty. By design routine poverty measurement hides the way gender influences experiences of poverty and inequality, and the default experience that shows up in data is the male one. This leaves decision-makers and change agents trying to act on gendered poverty without gendered data, and prevents us from ending poverty in all its forms.
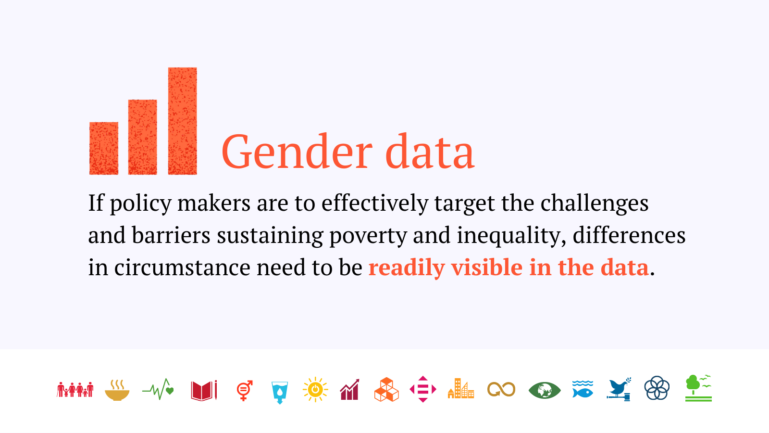
The data we need to make progress
Individual-level data
Another problem with measuring poverty of households rather than individuals, is that around one-third of global inequality lies within households. Or in other words, just because people live in the same household, it doesn’t mean they experience poverty and inequality in the same way. This means that global poverty and inequality are routinely underestimated by around one third. When the circumstances of individuals are not visible in data, it creates challenges to reduce inequality not just between households, but within them. This is a problem, but can be addressed by using individual-level measurements.
Measuring multidimensional poverty
Poverty isn’t just a lack of money, and it’s important that poverty measurements consider the material (food, water, sanitation etc.) and non-material (relationships, voice and time-use etc.) aspects of poverty. Poverty measurement must be multi-dimensional and consider a range of different aspects of a person’s life. This recognises that not all problems associated with poverty can be addressed simply by having a bit more money in your pocket.
Gender-sensitive data
Representation and visibility in data matter. Data that shines a light on gendered experiences of poverty and inequality are critical for justice and equality. Gender-sensitive data means collecting and analysing information about the areas of life that are often influenced by gender or gender norms such as unpaid work, time-use, voice, menstrual hygiene and access to contraception and family planning. This kind of data that is specifically designed to tell us something meaningful about gendered experiences can disrupt existing narratives and make space for gender-sensitive, evidenced-based policies and decisions.
Individual-level measurement of multidimensional poverty is essential to get the disaggregated data required to achieve Goal 1 – No poverty, Goal 5 – Gender equality, and Goal 10 – Reduce inequalities (among others). This type of data is also essential if we are to meet the overarching commitment to leave no one behind. We can’t see how overall progress is translating into outcomes for particular groups without the disaggregation of data that is enabled by individual level, gender-sensitive measurement.
And this information is important for decision-makers who have responsibility to allocate finite resources for greatest economic and social impact. There are real costs to maintaining the status quo. These costs have been normalised to the point of invisibility but the people who bear them include women, non-binary and gender-diverse people, as well as people with disability and many others who experience oppression and marginalisation. With finite resources being invested into data, continuing to collect gender unaware data is a lost opportunity to grow the availability of gender transformative data. In turn, this limits global progress to achieve the SDGs.
If we are going to ‘build-back better’, ‘leave no one behind’ and ‘end poverty in all of its forms’ – then we need data that is capable of identifying poverty in all of its forms and highlights inequality. With the right data to guide action on the SDGs we might just be able to get progress back on track.
A shorter version of the piece was first published on Pro Bono News.
Comments